Moving Retail Beyond the Barcode
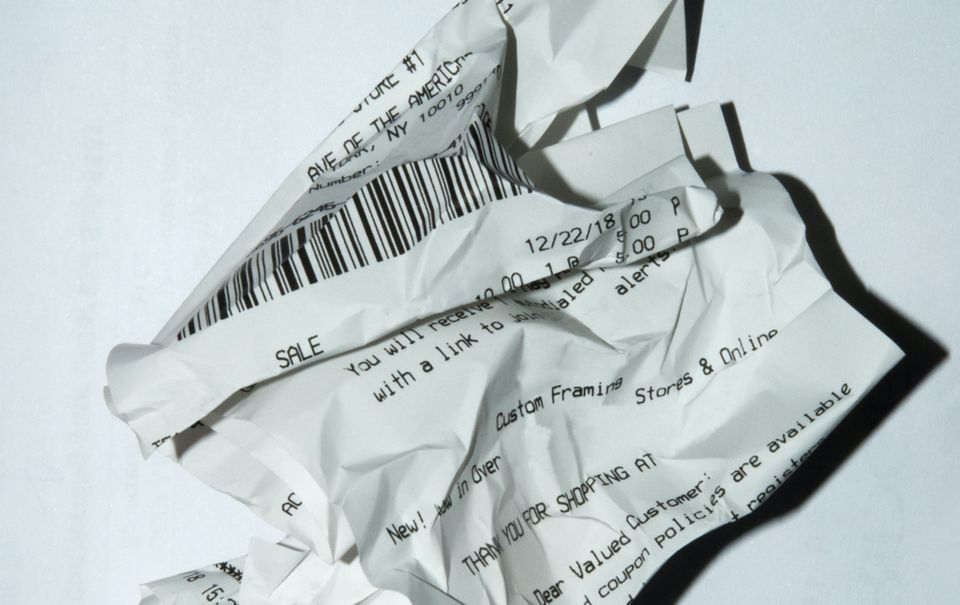
“The barcode is brilliant, cheap and reliable, but it has limitations that are hampering visibility of key cost-saving metrics. Intelligent cameras can illuminate these areas and deliver insights to many retail business units.”
A little history
The barcode is one of the more incredible inventions of the late twentieth century. It may not be as high in the pantheon as the personal computer or the cell phone, but it has arguably reshaped the world just as much. Plus, it passes Albert Einstein’s test that “if you can’t explain it to a six-year old, you don’t understand it yourself”.
The barcode was first imagined as a kind of visual Morse Code, which uses combinations of long and short beeps to represent letters and numbers. The beauty of the visual version is that it only has to represent numbers, so the long and short strips of black and white only need to have ten possible combinations. The actual story behind it’s invention is a fascinating series of twists and turns, brilliantly told here by the Smithsonian magazine. Suffice it to say that it took a long time, and a few major technological breakthroughs, to go from the first fingers-in-sand doodle on a beach in Florida to a pack of Wrigley’s gum being the first ever product scanned at a supermarket checkout, in the city of Troy, Ohio.
Let’s stick with Wrigley’s to explain exactly how huge the influence of the barcode has been. That first barcode scan took place in 1974 at a time when Wrigley’s gum had three flavours — the original pair of Juicy Fruit and Spearmint, both first concocted in 1893, and Peppermint, which was introduced in 1914. Astoundingly, these three products allowed William Wrigley to build Chicago’s first air-conditioned office skyscraper and take control of the local baseball team, renaming their field in the process. A pack of gum was chosen to be the first item to be scanned because it was so small that printing a useable barcode on it would be a newsworthy feat.
Since 1974, those three flavours have been joined by a ticker-tape parade of flavours and sub-brands — Freedent (designed not to stick to dental braces), cinnamon flavoured Big Red, sugar-free Extra, and on, and on, and on. Today there are more styles, flavours, pack-sizes and choices than could possibly have been imagined in 1974. And this is due in large part to the humble barcode.
Having accurate sales figures that could be reported quickly gave brands and manufacturers license to experiment with new lines and new products, building on the successful ones and quietly dropping the failures. Entire categories of products can come and go as brands use the data from barcode scans to invest in new trends and divest from dying ones.
The barcode has not only provided a playground for product experimentation and a more efficient checkout experience in the store, it also helped heighten product visibility all the way up the supply chain, massively reducing costs due to over-stocking or the losses due to going out of stock. The barcode is a cheap and dependable way to reduce costs and increase efficiency through manufacturing, logistics, merchandising and final sale of every packaged consumer good.
It’s worth remembering, though, that the reputation for low-cost reliability came on the back of huge research and development efforts around the world. Were it not for the miniaturisation of the laser (to the point where they could be built on a single microchip), the exponential growth of computer processing power to quickly read the data from the laser and turn it into actionable data, the millions of dollars invested in improving high-speed packaging printers, the barcode would probably have remained a dream doodled in the sand. Of course, a counter-argument here is that, for at least twenty years now, retail and supply chain technology companies have been trying to move away from the barcode and have not been able to come up with anything close to successful.
For all its usefulness, the barcode has a few significant limitations that are potentially holding back further leaps in operational efficiency. Readers can only scan one product at a time, for instance. Also, packages must be handled to turn the barcode towards the reader, taking two or three seconds per item for the average checkout operator. Also, a linear barcode only contains a small amount of data which can only be made useful with reference to other databases that contain product descriptions and price information. Queues at checkouts are due to the process of unloading, scanning and bagging that is required to accommodate these short-comings, but the barcode is so embedded into retail that it is extremely difficult to move away.
After the beep
RFID has been the most high-profile barcode-replacement candidate — a technology that has been touted as being on the verge of explosive growth and widespread adoption every year for at least the last fifteen years. The lack of breakthrough is due to it being something of a solution looking for a problem where the problem statement is “how do we fix the limitations of barcodes?” In the early days, manufacturers were slow to adopt unifying standards, which split the market in two. This significantly slowed adoption, as companies waited to see which technology would ultimately become the standard bearer. To this day, tag costs remain stubbornly high and readers are cumbersome. The potential benefits are undoubtedly compelling — multiple items can be identified simultaneously, making stock management a comparative breeze, and there is no need to point the tag at the scanner for the product to be identified. Ultimately, though, the benefits of greater efficiencies have not out-weighed the risks of re-engineering fundamental processes, particularly at the checkout.
A newer technology that could eventually do away with the need for barcodes altogether comes in the form of machine vision and image recognition. Like the long and meandering history of the barcode, machine vision has taken many twists and turns since the concept first emerged in the 1960s. Current applications rely on the emergence and development of various machine learning and deep learning technologies, as well as the physical improvements in digital camera technologies. Many instances also make use of the powerful processing now commonplace even in small computers. Some rely on high capacity networks and cloud-based storage and processing systems.
There are numerous applications in the retail industry around customer journey, such as people counting, dwell time measurement and analysis with some companies even touting the ability to track gestures in real-time to predict theft or other suspicious behaviour. However, tracking customers in such close detail quickly becomes problematic in terms of privacy, customer relations and business ethics.
A different set of applications leverage the efforts of the consumer packaged goods industry to make their products recognisable on the shelf. As consumers we have become so accustomed to quickly recognise brands and categories based on mixture of shapes, colours and typefaces. We are so immersed in this visual language that we can choose between detergent brands, breakfast cereals or kinds of cola almost effortlessly. At their simplest level, computer vision technologies work by making connections between objects with similar dimensions and colours , much like a shopper in a cereal aisle. With the right training data, these systems can learn to identify any of the 15,000–20,000 skus that a supermarket might sell with an accuracy of well over 95% and within fractions of a second. These kinds of figures very closely resemble the dependability of the barcode scanners of a few years ago and will only improve as the technology matures.
Loose produce is more problematic, although systems are becoming available that can reliably distinguish different types of apple, for instance. Some even claim to be able to tell whether a particular type of apple has been grown traditionally or organically.
The Value of Visibility
Product identification systems using machine vision can be used to generate stackable value cases for different business areas. For example, profit protection units can begin to accurately measure the value of products that are not scanned, either accidentally or maliciously, at the checkout. At the same time, cameras over checkouts can determine which products require repeated barcode scanning attempts, giving fast insight into how operational efficiency at the front end can be improved.
Analysing feeds from cameras in the aisles might be able to build a picture of how much product is being taken out of the store while avoiding the checkout entirely. In parallel, in-aisle cameras could also be used to give an always-on, real-time view of on-shelf availability.
Cameras above self-service checkouts can give an accurate picture of losses due to theft or errors, but can also be used to help customers act honestly by spotting errors and providing opportunities to self-correct. From a service perspective they can also help customers identify fresh produce without having to trawl through often confusing item look-up menus.
Miniature cameras can also be fixed to shopping trolleys, allowing shoppers to get accurate information about how much they are spending during their trip and possibly help them to avoid having to unpack and re-bag at the checkout. This same technology can also be used by staff to pick online orders for delivery or kerbside pick-up.
The great strength of machine vision solutions is that, in many cases, they can be implemented using existing in-store camera systems and can begin to return value without re-engineering processes or testing new customer journeys. The barcode is brilliant, cheap and reliable, but it has limitations that are hampering visibility of key cost-saving metrics. Intelligent cameras can illuminate these areas and deliver insights to many retail business units.