On Bias
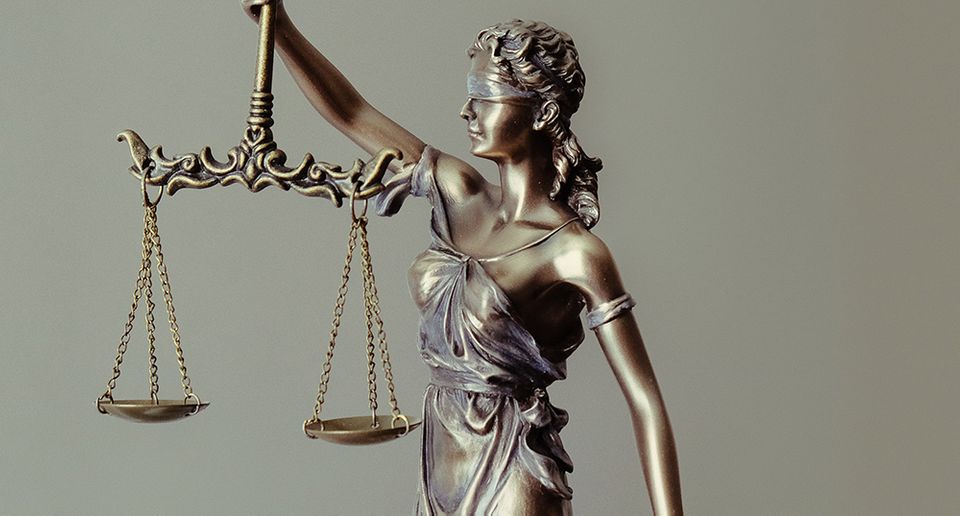
This article from VentureBeat raises many concerns about the technology that retailers are deploying in for shoplifting detection. While our systems are not mentioned in the article, it is important for us to be very clear on this subject - our loss prevention systems have been designed to be free of bias. Our models are trained only on real-world, in-store data - we have never had to use synthetic data of the kinds mentioned in the article because our systems were designed and built in partnership with major retailers. Furthermore, we never process personally identifiable information nor rely on facial recognition technology.
Our recommendation is that if your provider has any facial recognition components, or if you are unsure where their training data comes from, you have a long talk with them and then have a longer conversation with us.
This is an area that we take very seriously and we have written in the past about how the development of AI needs to go hand-in-hand with a robust and continually reviewed ethical framework.
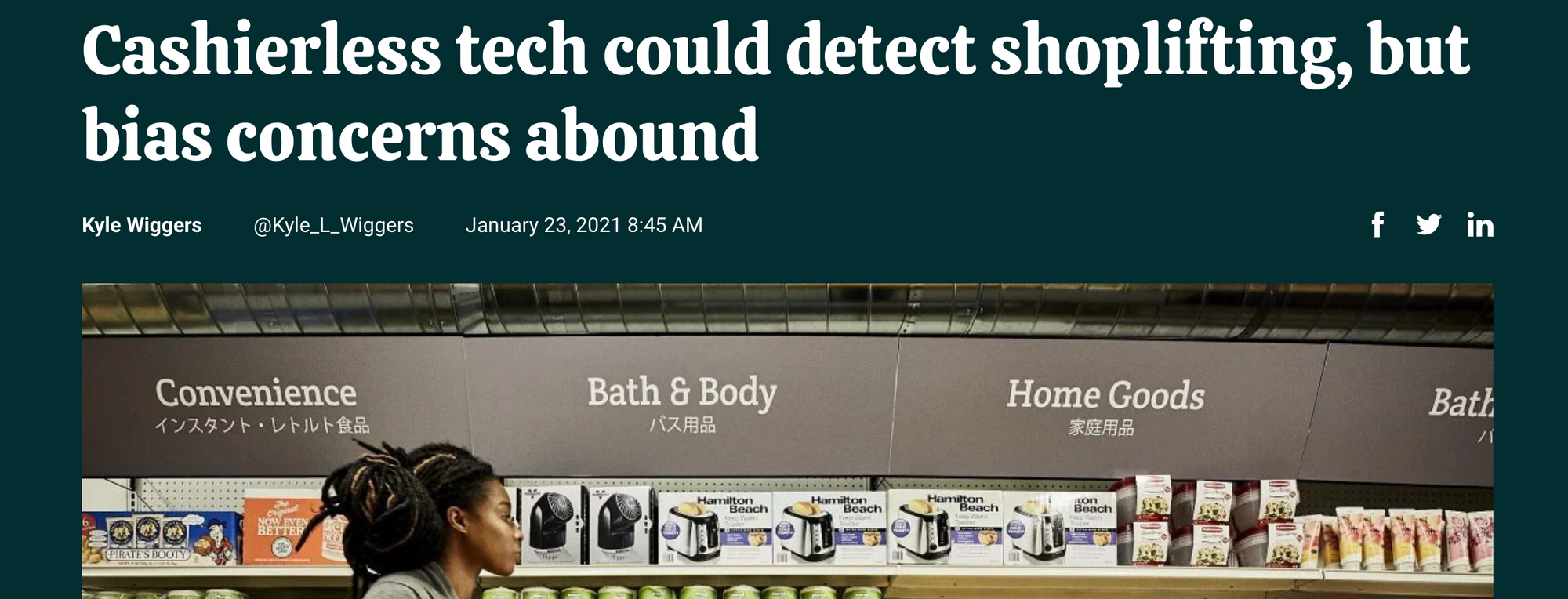
To briefly set out the issues in lay terms, AI systems work by identifying patterns within enormous data sets. Any biases in the data will in many cases be acted on, and can actually end up being amplified by the algorithms. For example, a facial recognition model developed at a university may use faces of past and present students as training data. If the students at the university have historically skewed towards being white or white-passing, any system built using this model is likely to misidentify people of colour. And this is not just an academic concern - there are many facial recognition systems being used today that are performing poorly precisely because of this problem. Indeed, the problems with facial recognition systems are so severe that many major tech companies have pulled support for them entirely.
Models trained on publicly available data sets are susceptible to biases hidden deep within the data. For instance, the widely used and much heralded GPT-3 language database has recently been shown to generate anti-Islamic text as a matter of course.
On occasion, the biases are more amusing. Janelle Shane, an optics researcher with a wealth of AI knowledge and a very readable book on the subject, points out how regularly systems are willing to predict the presence of giraffes without every knowing what giraffes are or why they might be present. Try for yourself with the Visual Chatbot, actually a pair of systems - one that will attempt to identify the contents of a photograph, and another that will chat with you about it. The image identification part works pretty well. The chat portion, however, has learned that if you ask how many of an object, say giraffe, are in the picture, the answer is likely to either be one or two. These are biases that the model has taught itself based on people using the system as it was intended to be used.
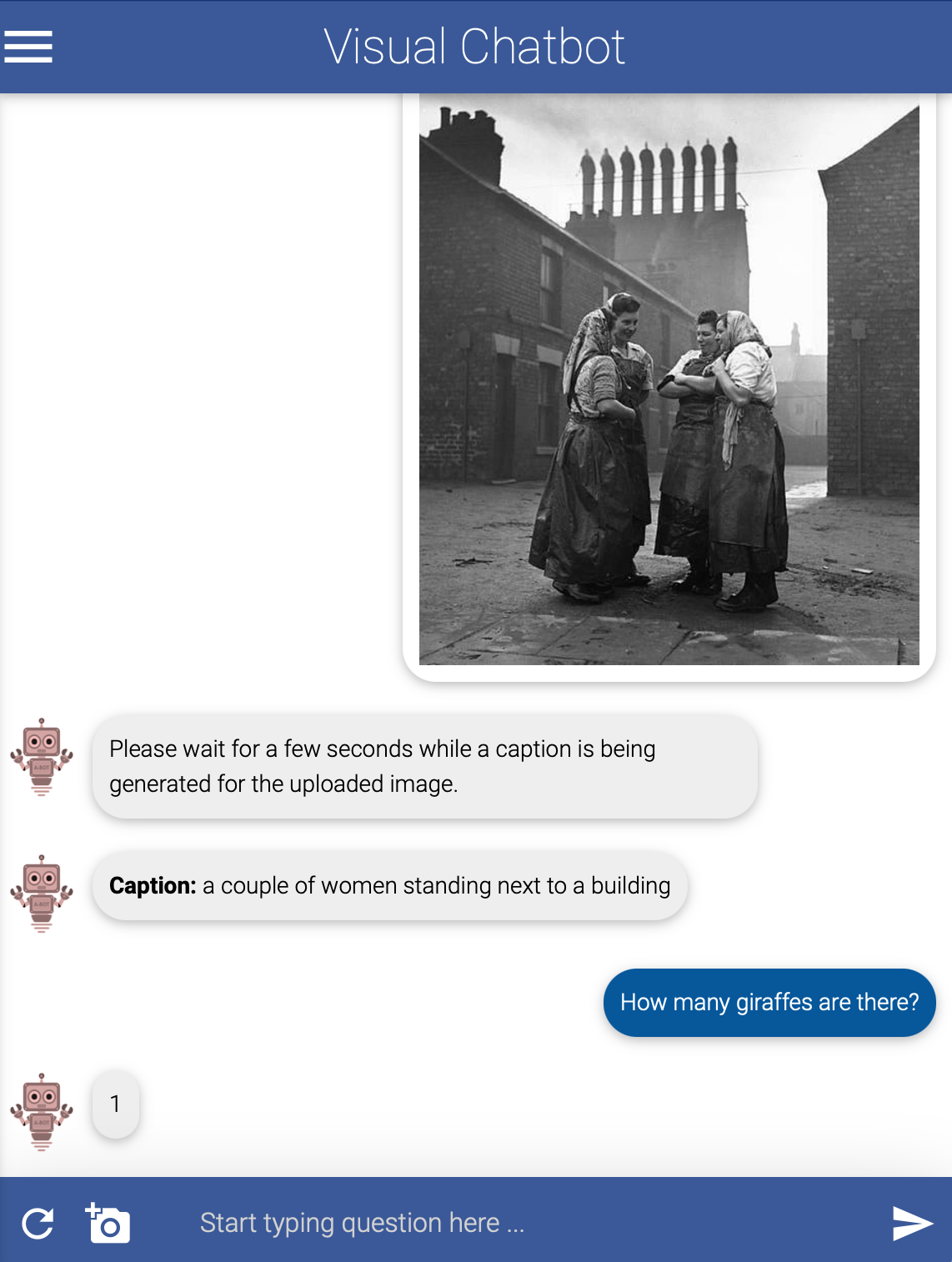
Biases, however they crop up, can make any AI system a little kooky at best, or damaging and disenfranchising to entire sections of society at worst. When systems are deployed, as ours are, in public spaces, extreme care must be taken to design the data architectures such that biases are not introduced from training data and that should they become evident, their sources can be identified and quickly remedied.