From CCTV to Cutting Edge AI: How Video Analytics has Transformed Self-Checkout Security
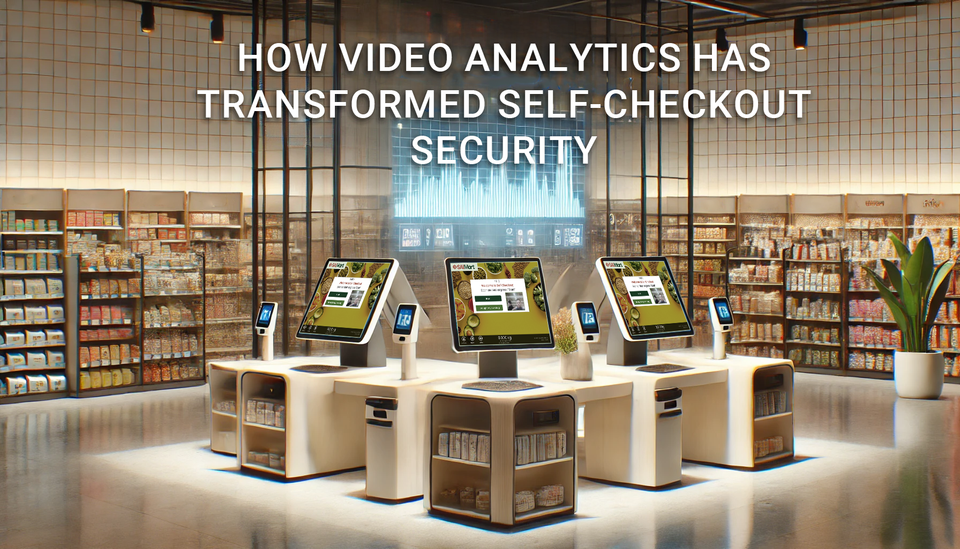
Video analytics has long held promise when it comes to identifying and preventing shrinkage at the self-checkout. The technology has undergone significant changes in the last few years, so much so that today's systems bear almost no comparison to their predecessors.
Let’s take a look at the evolution of video analytics and computer vision as a tool for stopping the misuse of self-checkouts, understand how things have changed and where they are today, and how things might adapt in the future.
The Earliest Implementations
Overlaying Scan Data on CCTV Feeds
When self-checkouts began to be embraced in the late nineteen-nineties and early aughts, artificial intelligence was still a computer-science pipe-dream. However, it was clear that offering customers the chance to scan and pay for their own shopping brought with it the potential for misuse. The solution at the time was to take an existing technology – a human looking at CCTV screens – and provide them a way of checking what was being scanned. The CCTV interface super-imposed a description of the scanned product onto the feed from a nearby security camera.
While these systems could effectively address a small proportion of the losses, they were only as reliable as the operator and their ability to focus on multiple screens simultaneously.
Pros | Cons |
---|---|
Better than doing nothing | Additional open-to-close labor cost |
Inaccurate and prone to human biases | |
Doesn’t scale for more SCO |
Assisted AI
Hybrid Computer-Human Decision Making
The main shortcoming of the CCTV Interface was the problem of having a human watching multiple video feeds and making an accurate assessment of complex customer behaviors. As such, accuracy was low and lots of loss went undetected. An obvious fix was to replace the person with such artificial intelligence capabilities as were available in the late twenty-tens.
From today’s vantage point, these early AI systems were limited in their capabilities. They were able to identify some loss events, although accuracy was low. By taking a lead from their technological predecessor, these incidents were shown to a human to be validated or ruled out remotely. Footage of validated incidents could then be sent back to the store for actioning.
Pros | Cons |
---|---|
Better than human-only monitoring | Lots of false alerts |
Still incurs staffing costs | |
High latencies |
Autonomous AI
Computer-only Decision Making
With refinements to computing techniques, the first autonomous AI systems began to emerge soon after their assisted predecessors. These took away the need for human event-validation and were able to make decisions using computing power alone. This step-change in processing requirements could be achieved using graphics-processing units (GPUs) which were becoming commonly available. While this drove up the cost of the hardware the solutions ran on, they were able to identify many more cases of loss than previous generations, which went some way to balancing things up.
The self-checkout corral can be a visually complex place in any store, so unsurprisingly these systems sometimes struggled to cope. Customers bring many objects into a store with them such as bags, purses, cell phones and car keys. Add in the movement of products, carts and baskets and the confusion rises exponentially. Without the comfort-blanket of human validations, these autonomous systems were prone to assume that personal items should be paid for at the checkout, and therefore interrupted customers unnecessarily. In some cases, accuracy could be improved with additional investment in dedicated high-spec cameras and the provision of constant light-levels, but this made costs prohibitively high.
Pros | Cons |
---|---|
Able to operate close to real-time | Expensive due to GPUs |
Customer frustration with false alerts | |
May require dedicated cameras and lighting |
Efficient AI
Customer-centric Decision Making
Today’s systems are much more efficient than earlier generations. With careful design and development, they run on standard edge-processors that don’t need GPU acceleration, even when monitoring forty self-checkouts. In many cases, they can leverage existing cameras, including those that are mounted on ceilings, without any need for speicalised cabling or lighting. This makes the overall solution affordable, but also requires less disruption in stores and avoids the need for obtrusive camera placement which can harm customer sentiment.
Advanced techniques and smart AI modelling mean platforms today can understand all of the visual chaos of retail, identifying all of the behaviors that lead to loss without interrupting customers for bringing their car keys with them. They can also adapt to a retailer’s specific operating models and workflows by nudging customers to self-correct errors or by alerting colleagues to assist as well.
Pros | Cons |
---|---|
Cost effective | |
Highly accurate | |
Cover all loss causing scenarios | |
Leverage existing infrastructure |
What does the future hold?
AI is an enormous growth area and the number of ways it touches our lives is growing every day, especially in the fields of computer vision and video analytics. Multi-modal systems – in other words, systems that can perform multiple tasks from a single code-base – are already emerging. At the self-checkout, this could see loss prevention, age verification, and produce identification running from a single platform, with the flexibility to build-in new use-cases as they emerge.
About SAI
As a leader in computer vision technology, SAI Group delivers cutting-edge, multi-modal AI solutions into retail environments. Using a unique platform approach, its technology uses existing camera systems to target losses, increase store safety, and underpin operational efficiencies.
All solutions are built from the ground up to ensure the highest levels of security and data protection, respecting the privacy expectations of the public and operating to stringent ethical standards while delivering substantial value to our clients. Globally, SAI monitors millions of transactions per day, protecting the revenues from tens of millions of product sales and hundreds of millions of customer interactions. Its models also accurately identify anti-social behaviour, aggression and violence, helping to de-escalate situations with real-time interfaces to security officers and operations centres.
Get your team together and join us for a look at the most comprehensive video analytic solution that top-tier retailers around the world rely on.